Mayo Clinic researchers have recently introduced a groundbreaking class of artificial intelligence (AI) algorithms known as hypothesis-driven AI, representing a departure from traditional models that rely solely on data learning.
In a review published in Cancers, the researchers highlight that this novel AI approach offers a transformative method for leveraging extensive datasets to unravel the complex origins of diseases like cancer and to enhance treatment strategies.
Senior author and co-inventor Hu Li, Ph.D., a Mayo Clinic Systems biology and AI researcher in the Department of Molecular Pharmacology and Experimental Therapeutics, underscores the potential of hypothesis-driven AI:
“This heralds a new era in developing targeted and informed AI algorithms to address scientific inquiries, gain deeper insights into diseases, and guide personalized medicine. It holds promise in uncovering insights that conventional AI may overlook.”
Traditional AI is predominantly employed in tasks such as classification and recognition (e.g., facial recognition) and increasingly in generative tasks like text generation.
However, these algorithms often do not integrate existing scientific knowledge or hypotheses, relying instead on vast, unbiased datasets which can be difficult to procure.
Dr. Li emphasizes the limitations of conventional AI, noting that they hinder flexibility in AI methodologies, particularly in knowledge discovery-driven fields such as medicine.
“The lack of integration between existing knowledge and hypotheses can lead to suboptimal outcomes.
AI models may produce results without the thoughtful input of researchers and clinicians, resulting in what is commonly referred to as the ‘garbage in, garbage out’ problem,” explains Dr. Li.
In contrast, hypothesis-driven AI aims to integrate scientific understanding directly into the design of AI algorithms.
For instance, in cancer research, this involves incorporating known genetic variants and gene interactions into the algorithm’s framework.
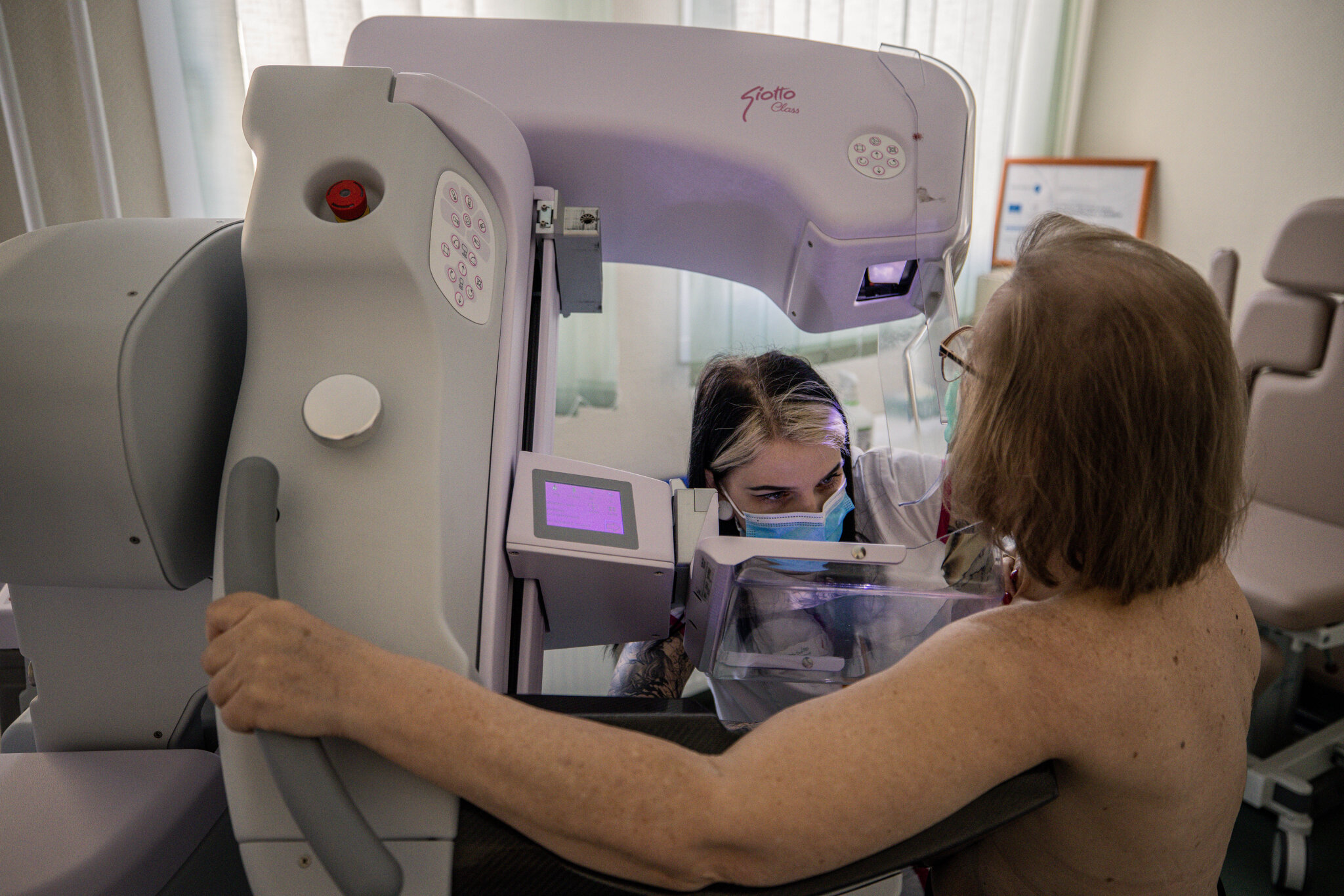
This approach enhances the interpretability of AI outputs and facilitates the formulation of testable hypotheses critical for advancing medical knowledge.
Daniel Billadeau, Ph.D., a professor in Mayo Clinic’s Department of Immunology and co-inventor of the study, underscores the potential of hypothesis-driven AI in cancer and immunology research:
“This innovative AI approach offers avenues to better understand the intricate interactions between cancer and the immune system.
It not only allows for hypothesis testing but also has the potential to predict and explain patient responses to immunotherapies.”
The research team envisions broad applications of hypothesis-driven AI across cancer research, including tumor classification, patient stratification, cancer gene discovery, drug response prediction, and analysis of tumor spatial organization.
Key advantages of hypothesis-driven AI highlighted by the researchers include its targeted focus on specific hypotheses or research questions, leveraging of existing biological and medical knowledge, enhanced interpretability of results compared to traditional AI, and reduced data and computational requirements.
Dr. Li acknowledges challenges such as the need for specialized expertise in developing these algorithms and the potential for bias.
However, he emphasizes that hypothesis-driven AI represents a significant step forward in medical research.
“While there are hurdles to overcome, hypothesis-driven AI has the potential to advance medical research substantially, leading to deeper insights and improved treatment strategies.
It could pave the way for enhancing treatment regimens and outcomes for patients,” concludes Dr. Li.